Japanese / English
U-Net based mitosis detection from H&E-stained images with the semiautomatic annotation using pHH3 IHC-stained images
Mitosis is one of the steps in cell division, a proliferative process to create new cells, and thus the number of mitotic figures represents the proliferative activity of the cell. Therefore, the number of mitotic figures is an important prognostic factor in the pathological diagnosis of cancer. The examination and counting of mitotic figures are performed manually by pathologists, which requires not only specialized knowledge but also takes a great deal of time and effort. Therefore, research on detection methods using deep learning, such as convolutional neural networks (CNN) and YOLO, has been actively conducted to automatically detect mitotic figures from images of Hematoxylin & Eosin (H&E) stained pathology specimens. However, the morphology of mitotic images is diverse, and the detection performance of conventional methods is still limited. In addition, the resolution of WSI is very high, so efficient methods are desired.
In this paper, we make the following three proposals in response to the above issues;
- The variation of the images in currently available datasets is insufficient to reflect the diversity of mitotic figures in the machine learning model. It is necessary to annotate mitotic images in dataset creation, but manual annotation is time-consuming and error-prone. In this study, we develop the automated annotation technique by utilizing pairs of H&E and phosphohistone H3 (pHH3) stained images of the same tissue. pHH3 specimens can detect the presence of a target antigen (pHH3) that identifies cell mitosis. The proposed method uses the tiling-based alignment of Whole Slide Images (WSIs) of H&E and pHH3 staining and applies a novel filtering method for fast and accurate labeling. The system successfully generates a dataset directly from the H&E and pHH3 stained image pairs, and we experimentally demonstrate that this dataset is effective as a data extension for model learning.
- As a new method for detecting mitotic images by deep learning, we propose a U-Net-based mitotic detection model (GaussUNet) trained with labels based on a two-dimensional Gaussian distribution. The Gaussian distribution is assigned to each center-of-gravity in the mitotic figure, resulting that the pixels closer to the center are learned with higher weight and the pixels farther from the center are weighted lightly. This process enables highly accurate detection with relatively simple processing.
- channel, a background channel, and a mitosis-look-alike (MLA) channel. The MLA channel is used to train the model separately. In previous work, the MLA annotation was given by pathologists. In contrst, this paper achieves improved model performance by adding false positives (FPs) obtained from the trained model as MLAs to the training data without a manual annotation.
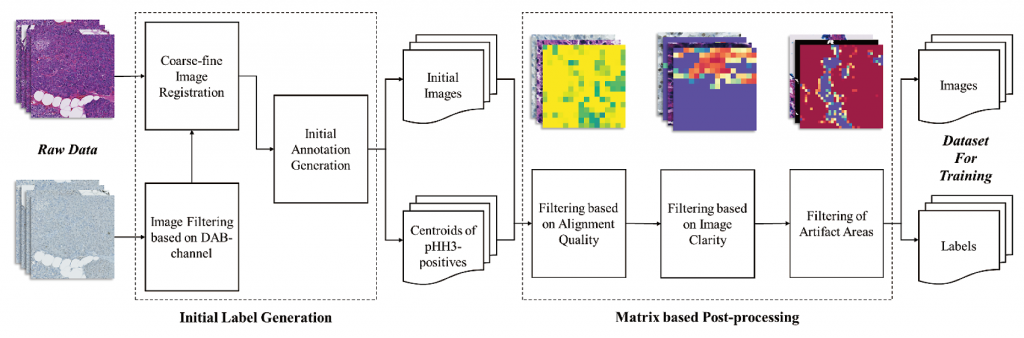
Fig. 1 The semi-automatic dataset generation algorithm overview
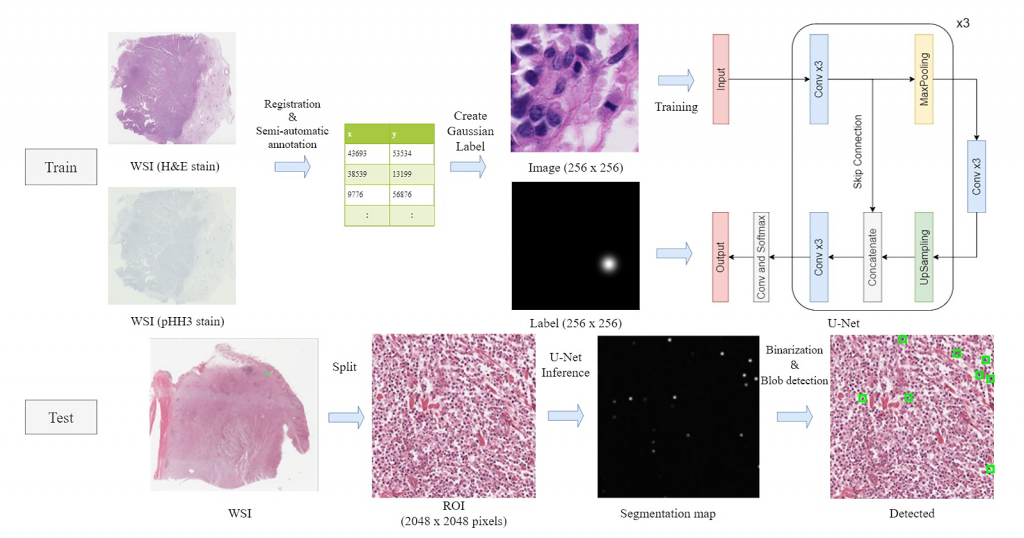
Fig. 2 U-Net-based mitosis detection method overview
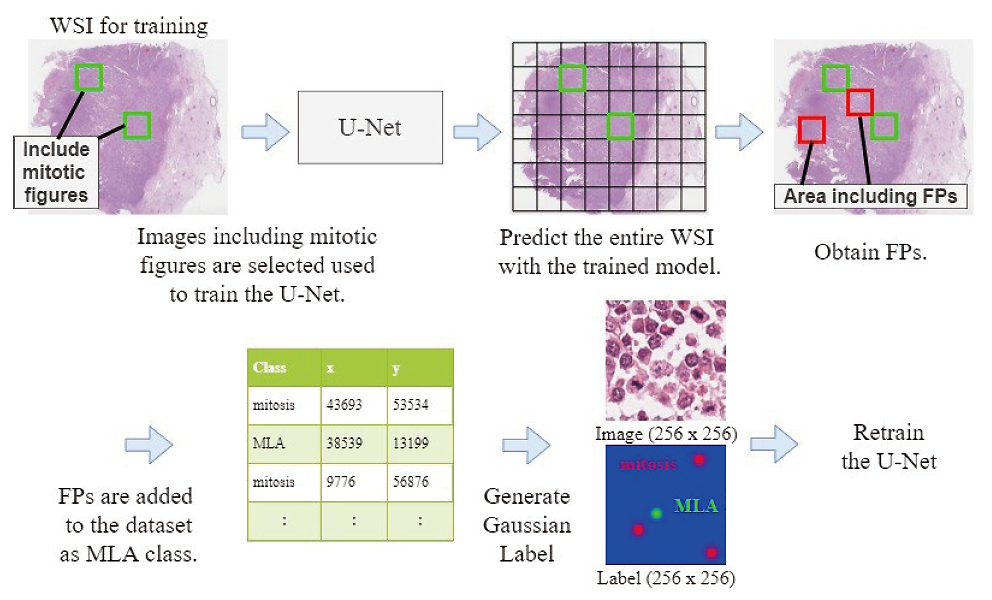
Fig. 3 Re-train the U-Net using FPs as MLAs.
Publication
[1] Jiaming Li, Toshiyuki Adachi, Saori Takeyama, Masahiro Yamaguchi, Yukako Yagi, “U-Net based mitosis detection from H&E-stained images with the semi-automatic annotation using pHH3 IHC-stained images,” Proc. SPIE 12032, Medical Imaging 2022: Image Processing, 120322J (4 April 2022); https://doi.org/10.1117/12.2612815
https://www.oid.ict.e.titech.ac.jp/publications/U-Net_based_mitosis_detection_from_HE-stained_images_with-letter.pdf
====
This is collaborative work between Memorial Sloan Kettering Cancer Center, USA, and Tokyo Institute of Technology, Japan.
This work was conducted in part under the project JPNP20006, subsidized by the New Energy and Industrial Technology Development Organization (NEDO).